Artificial Intelligence • August 2nd, 2023
In today’s digital landscape, where users are overwhelmed with information and choices, personalization and customization have emerged as the pillars of a compelling user experience. Enter Artificial Intelligence (AI), the driving force behind the ability to tailor products, services, and content to individual preferences and needs. This article embarks on a deep dive into how AI empowers personalized user experiences, unveiling the mechanics of recommendation systems and content customization that enhance engagement and user satisfaction. Welcome to the era where AI crafts unique journeys for every user, reshaping the way we interact with technology.
The Rise of Personalization
Personalization is not a novel concept, but its implementation has reached unprecedented heights with the advent of AI. At its core, personalization aims to create experiences that resonate on an individual level, making users feel understood and valued. AI achieves this by harnessing vast amounts of data, processing it in real time, and delivering tailored recommendations, content, and interfaces.
Recommendation Systems: The Magic of Personalization
At the heart of AI-driven personalization lies recommendation systems. These systems analyze user data, including past behavior, preferences, and interactions, to make informed predictions about what users might like or find valuable. The mechanics are complex, involving algorithms that continuously learn and adapt to user feedback. Whether it’s suggesting movies on a streaming platform, products in an e-commerce store, or articles on a news website, recommendation systems are omnipresent, driving user engagement and retention.
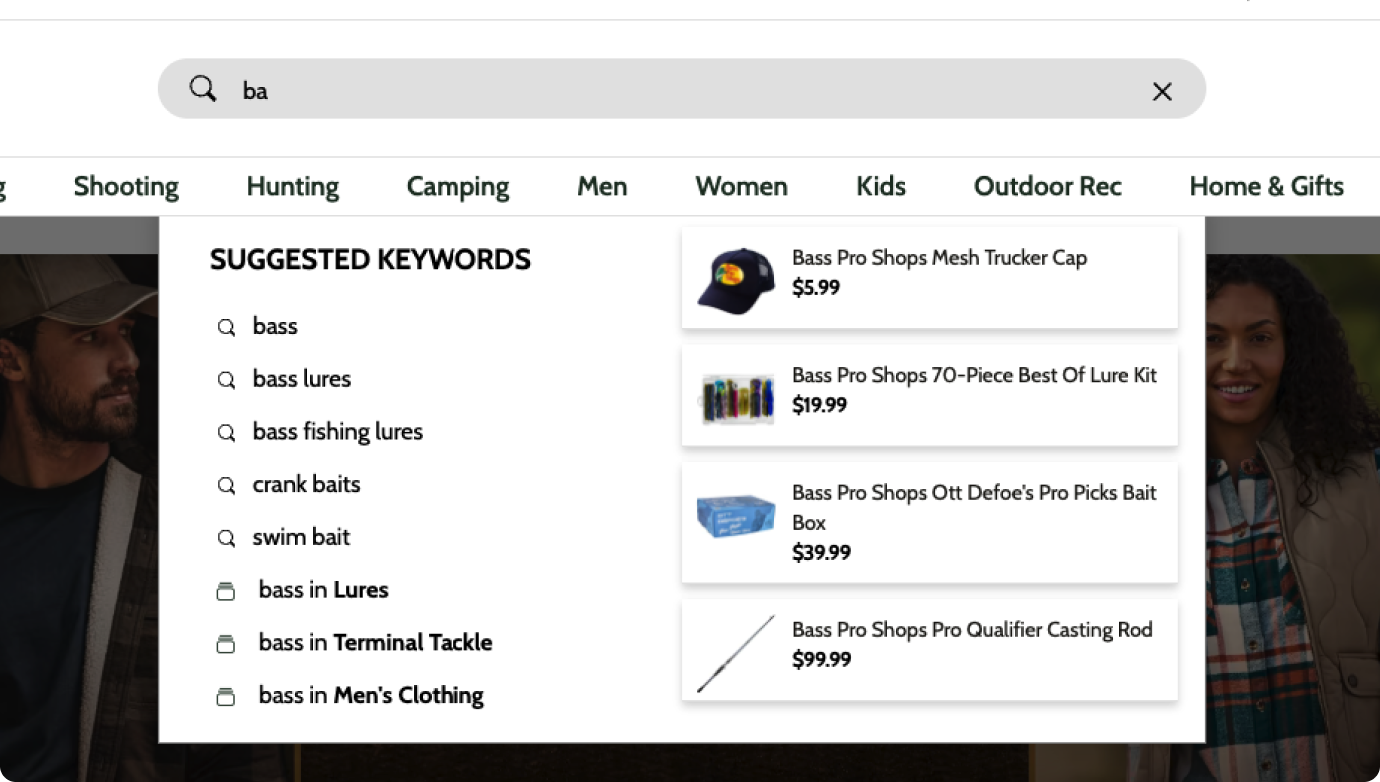
The AI-powered platform Coveo can predict what people need, before they even know they need it
The Inner Workings of Recommendation Algorithms
To understand the mechanics of recommendation systems, it’s essential to explore the two primary types of algorithms they employ:
Collaborative Filtering
Collaborative filtering relies on user behavior patterns and preferences. It identifies users with similar tastes and recommends items that those similar users have interacted with. There are two main collaborative filtering approaches: user-based and item-based.
- User-Based Collaborative Filtering: This method identifies users who exhibit similar behavior and recommends items based on what those like-minded users have chosen.
- Item-Based Collaborative Filtering: In this approach, the system recommends items similar to those the user has shown interest in, based on item-item similarities.
Content-Based Filtering
Content-based filtering, on the other hand, relies on the attributes of items and a user profile. It recommends items that are similar in characteristics to those a user has shown interest in.
Beyond Recommendations: Content Customization
While recommendation systems focus on suggesting items, content customization takes personalization a step further by tailoring the actual content itself. AI-driven content customization involves dynamically altering text, images, or other media to match a user’s preferences or context.
For instance, an e-learning platform might customize course content based on a student’s learning pace and interests. Similarly, a news website can personalize headlines and articles to align with a user’s reading history and preferences.
The Impact on User Engagement
The implementation of personalization and content customization is not just about offering a personalized experience; it directly affects user engagement. When users encounter content that aligns with their interests, they are more likely to:
- Spend more time on a platform.
- Interact with content, whether through likes, comments, or shares.
- Make purchases or consume more content.
Challenges and Ethical Considerations
While AI-driven personalization brings tremendous benefits, it also raises important challenges and ethical considerations:
Data Privacy and Security
To deliver personalized experiences, AI relies on user data. Safeguarding this data against breaches and ensuring user privacy are paramount concerns. Striking the right balance between personalization and data protection is an ongoing challenge.
Filter Bubbles and Echo Chambers
Over-reliance on personalization can lead to filter bubbles, where users are exposed only to content that aligns with their existing beliefs and interests. This can reinforce bias and limit exposure to diverse perspectives.
User Consent and Transparency
Users should have control over the extent of personalization applied to their experience. Transparency about how AI operates and the ability to opt out of personalized features are essential for maintaining trust.
The Future of AI-Powered Personalization
As AI continues to advance, the future of personalization holds exciting possibilities. AI may move beyond traditional recommendation systems and content customization to offer real-time, context-aware personalization. Imagine a world where every interaction with technology adapts seamlessly to your needs and preferences, providing a truly tailored experience.
Conclusion
AI has ushered in a new era of personalization and customization, where every user is treated as an individual with unique preferences and needs. Recommendation systems and content customization algorithms lie at the heart of this transformation, reshaping how we interact with digital platforms. While challenges exist, the potential for enhancing user engagement and satisfaction is immense. As AI continues to evolve, the future promises even more personalized and meaningful experiences, revolutionizing the way we engage with technology.
If you enjoyed reading this article, please share it with a colleague or friend, and join me next week to learn about Design Optimization with AI.